When thinking about all the readers of the article, we’ve realized that there will probably be two entirely different groups - the ones completely new to the idea of artificial intelligence in recruiting, and the experts who are already leveraging their AI usage. However, regardless of which group you belong to, we invite you to keep reading the blog and get to know the evolution process of AI in recruitment on some real life examples.
AI recruitment pioneers
It’s been a long way since 2014 and the infamous Amazon case in which the company mistakenly created a biased recruiting software that would end up repeating human mistakes by discriminating against women. Luckily, the company has abandoned the project and it became one of the greatest learning lessons for using AI in recruitment. So, what really happened at Amazon?
While the company tried to be the pioneer in automating the recruitment process, the way they collected data for making the decisions was flawed. The idea behind AI-powered technology is that for it to make future decisions, it needs to be fed with large amounts of data. Therefore, this leads to a logical conclusion that the quality of data is a crucial part of the process. However, Amazon made a mistake here. The way they provided data for their software was by feeding it with recruitment information of the company from the previous 10 years of hiring. The outcome was biased against women and showed that just like a human makes mistakes, the software can do the same. For many who didn’t take a close look at the issue, this caused mistrust in using new technologies for such important tasks.
However, big attention to this case was drawn by the entire industry, and here are a couple of good takeaways that helped prevent the same mistakes in the future:
Machines are not the one producing the bias: Machines are trained by humans. If the data that the machine consumes is based on biased decisions of humans, the machine has no other way to perform the tasks than to repeat the same mistakes. This mistake created a new challenge; how do we create the machine as immune to human mistakes as possible?
The importance of data: In the Amazon case, the company was using only the data from its previous hirings. Even though Amazon is an enormous company, this data still isn’t enough to feed the software for the sake of diversity.
Making future decisions based on past events: Finally, one of the most important mistakes in the Amazon case was basing future decisions on the past 10 years of recruitment data at the company. Within those years, the policies, hiring trends, and procedures changed drastically. The use of such data for building the recruitment software of the future represented only a setback for the company.
Always own your mistakes: While developing a complex piece of technology that will make future decisions for your company, it is a necessity to analyze every step of the way and to be transparent with your mistakes. Mistakes will happen, but only truly owning them can be a good learning lesson for the future. In the Amazon case this can mean deleting the elements of the CV that are subject to biases and training software for the new conditions.
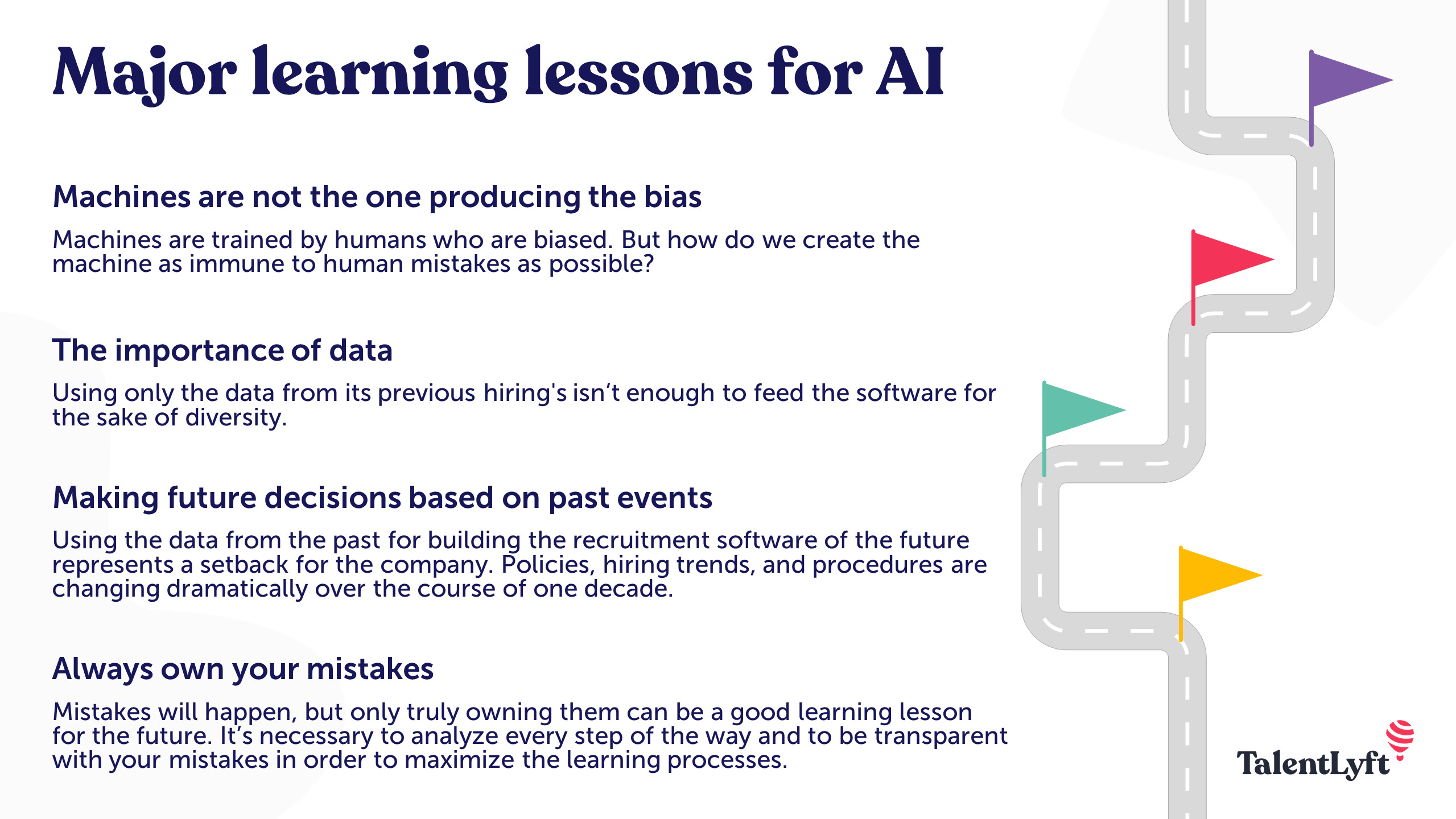
The second wave and the era of keywords
Learning from Amazon’s mistakes gave a great deal of confidence for others who attempted to create recruitment software of the future. This created an entirely new way of screening CVs - by using keywords.
This means that now the software was able to screen through a large amount of CVs, searching to find certain keywords that were previously set. At the first glance, this way of conducting CV parsing was amazing. However, it didn’t take long for recruiters to realize that some candidates found a way to cheat. By simply disguising a bunch of relevant keywords in their CVs and writing them in a white font, candidates were now able to position themselves way up high in the recruiter’s list regardless of their actual competencies.
Besides people finding loopholes and ways to trick the software, another big problem arose. There are countless ways in which people with similar skill sets can name and describe their education, previous work experience, or soft skills.
For example, three different people from Free University Berlin can use different ways to name it in their CV; the first one using the full name in English, the second one using a short name - FUB, and the third one using the original name in German - Freie Universität Berlin. And this is just the name of the University, try imagining what happens with the software when people start naming their soft skills. Ultimately, the software wasn’t yet capable of understanding the similarities and differences between synonyms, homonyms, or difficult concepts.
In order to address these issues softwares began using something called Ranked Retrieval systems that had already been available for many years but used for different purposes (such as for Google search). In this context, the system works in a way that it analyses a plain text query a user writes into a query box (instead of making the user learn how to code information into booleans) and attempts to construct a query automatically. The results are then ranked in order of relevance to the query depending on the context and number of times in which terms appear in documents.
While this technology simplifies the process for the recruiters by not requiring the knowledge of complex Boolean syntax, the end result becomes less accurate and loses the power that Boolean queries provide. This can result in losing relevant candidates that end up not being discovered at all or being too low in recruiters list.
Semantic similarity is the way to go
Thanks to all the mistakes along the way, AI has been developing tremendously which paved the way for deep machine learning techniques to start overtaking the AI recruitment processes. The newest semantic-based AI can eliminate all the previous mistakes and help find the best candidates faster. When software uses semantic similarity for natural language processing as a way to read through a high volume of CVs here is what happens.
Based on the enormous amount of CVs that the software reads through, it starts learning the patterns and most importantly understanding the content of those CVs. This goes way beyond simply recognizing certain keywords. Most importantly, thanks to semantic similarity, the language gap between the recruiter's job descriptions and candidate's CVs can be overcome.
However, it is important to mention that it is not a single tool that leads to a revolution in AI, but that it’s the combination of multiple technologies which aim to minimize previously mentioned issues. For instance, AI technology uses models that are generally trained for understanding a certain language (often used English). By taking such model and training it to understand a certain domain (such as the domain of human resources) the technology learns the HR related vocabulary and is then capable of extracting certain words, terms and expressions and matching them with a specific level of expertise - leading to a final act of matching CVs with the job requirements. The model is trained based on predetermined characteristics that would lead to the most accurate way of matching.
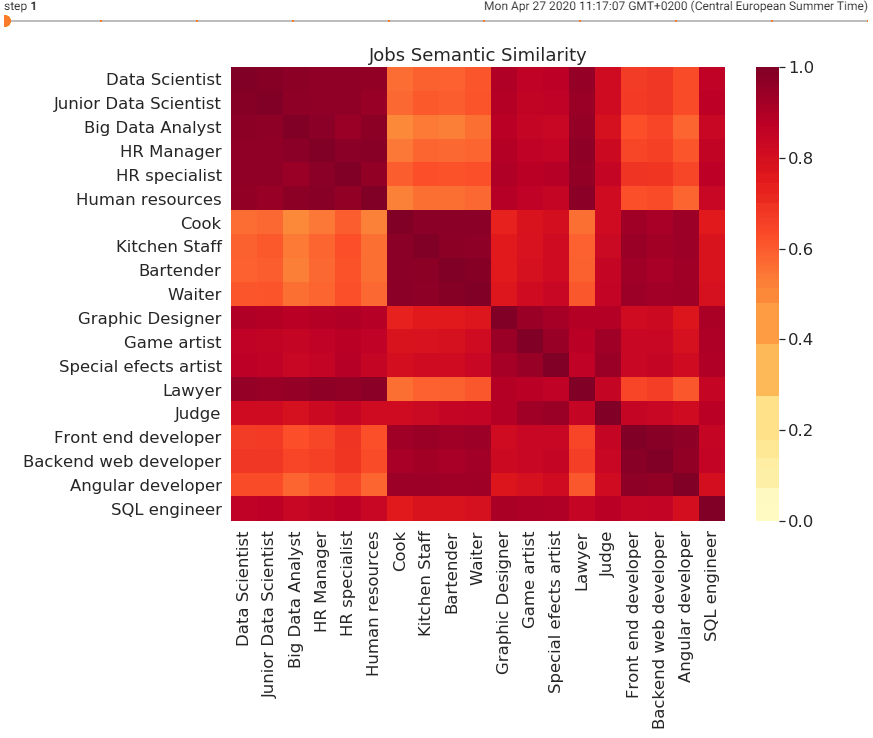
This way the task of imputing all relevant synonyms manually or based on keywords becomes redundant since the software learns its own patterns and ways of attaching some words to another and the opposite. When put simply, the three different people from the previous paragraph who are listing their education at Free University Berlin by using different expressions can now be understood by the software.
Furthermore, a technology called Contextual Validation is used to understand the context and frequently used terms to accurately place a candidate with a specific job requirement. This way resume parser analyzes which terms the candidates are using to refer to their skills, and which terms are merely referring to incidental context, which ultimately increases the accuracy.
Finally, for the benefit of ranking candidates, the technology called linguistic analysis/resume parsing and ranking can be used. This technology is capable of ranking the candidates based on not only a particular skill of the candidate but also on the level of experience of using that skill. One of the technology's key features enables recruiters to have their candidates ranked and in order so that they are always sure that the highest rank belongs to the most relevant candidates and opposite.
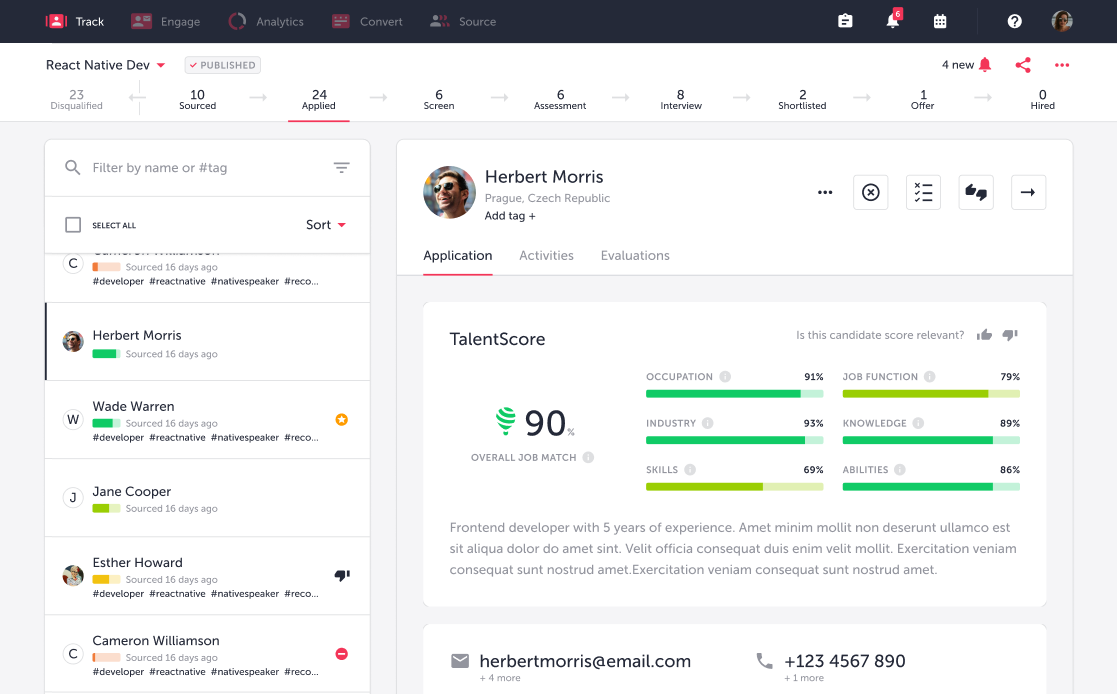
(a preview of TalentLyft software)
AI needs us to keep learning
Finally, it’s been a long way for AI since its beginnings. However, there is no sign of slowing down. With every day and every feedback, AI, the developers, and the recruitment industry are getting better at understanding the issues and looking into the needs of the entire recruitment process.
Instead of sitting back and enjoying the ride, it’s time for all of us to embrace the learning mentality and taking part in creating the technology of tomorrow!